As a long term partner of IntelliHQ, a Queensland AI Hub consortium member, the TechConnect team was alerted to the Queensland AI Hub Medical datathon to be held over 2 weeks in June 2020. TechConnect is an AWS advanced consulting partner with a data science team whose experience spans from beginner to expert. As such, the event was seen as an opportunity to mentor the team while contributing to a pressing need – the application of Artificial Intelligence (“AI”) technologies in the medical domain. With an emphasis on COVID-19 and a pre-conceived project centered on improving the prioritisation of chest x-ray data, the team was highly motivated to donate their time and deliver a proof of concept implementation.
After several webinars setting the scene for the datathon, participants were allocated to teams and each team began introductions and brainstorming their solution. Each team was comprised of subject matter experts volunteering from multiple departments within Queensland Health, academia and other private medical organisations in addition to technical specialists – both in Cloud and Data Science. The TechConnect team were allocated to the Amazon Web Services 2 (“AWS-2”) datathon team. Within the AWS-2 team we had an ex-software engineer (now working in the emergency department), an emergency department nurse, a physicist (specialising in x-ray and other imaging devices) and researchers with experience working with medical imagery. To support all teams, each team was assigned a support engineer from the cloud provider on which they were building. After introductions and brainstorming the team split into two primary factions – the pitch team and the model building team.
Within the model building team the emphasis was on collecting the current state of the art datasets and classifications with the intent to replicate the results of those existing machine learning models. Having done so, the classes were redefined to match our different use-case (that of augmenting the radiologist by providing an urgency score rather than predict particular diagnoses) and the models were re-built to the use case. Some of the team worked on data preparation – clean data is essential for success – while others investigated using the models to highlight the regions of the image that most influenced the classification. Multiple approaches to model building were utilised – using custom compute instance types and the hosted machine learning platform AWS SageMaker. Both were a necessity due to differing skill sets within the team and the limit on compute instances available to work with. Multiple deep learning libraries and architectures were tested throughout this process, again, aligning to the skills of the most experienced data scientists in the team.
The pitch team focussed on refining the message, sourcing statistics on the magnitude of the problem, understanding and comparing our approach to other solutions in the marketplace and proposed the potential impacts of solving this challenge. A short animation describing the patient journey and patient outcomes was embedded into the pitch. This was crucial to put the patients perspective front and centre with emphasis on the costs of not solving the problem; this presented the problem in a way that statistics never could. The time allocations for sharing our vision with the judges were small and so significant efforts were spent refining and sharpening the deck to fit the constraints while not missing important details.
The key motivations for utilising artificial intelligence to rank the urgency of review for the chest x-rays included:
- Worldwide 2,000,000,000 chest x-rays are taken
- 300,000 patients in the NHS are waiting longer than 30 days for the results of their chest imagery
- 200,000 chest x-rays went unreported at an Australian hospital prior to them outsourcing this work in 2014.
- Emergency department doctors are making decisions on the returned images prior to the radiologists completing their reporting due to the turn-around times on the reports. Thus, the radiologists efforts are not taken into consideration during diagnosis.
It is the teams strong belief that enabling the radiologists to focus on the few images with abnormalities will go a long way to alleviating the issues the current system operates with. Further, the reduced cost of screening opens up opportunities to utilise these same imaging technologies in poorer and lesser developed regions of the world. Given the growing interconnectedness of nations, being able to scale these kinds of technologies makes for a healthier global population, both in the regions for which this technology will be new and those countries that already use chest x-rays extensively.
With hours to spare, the modelling team finalised their results and were able to incorporate the basic accuracy measures they were able to attain. Some imagery demonstrating the models ability to identify the key regions in the lungs that deserved further investigation are shown below.
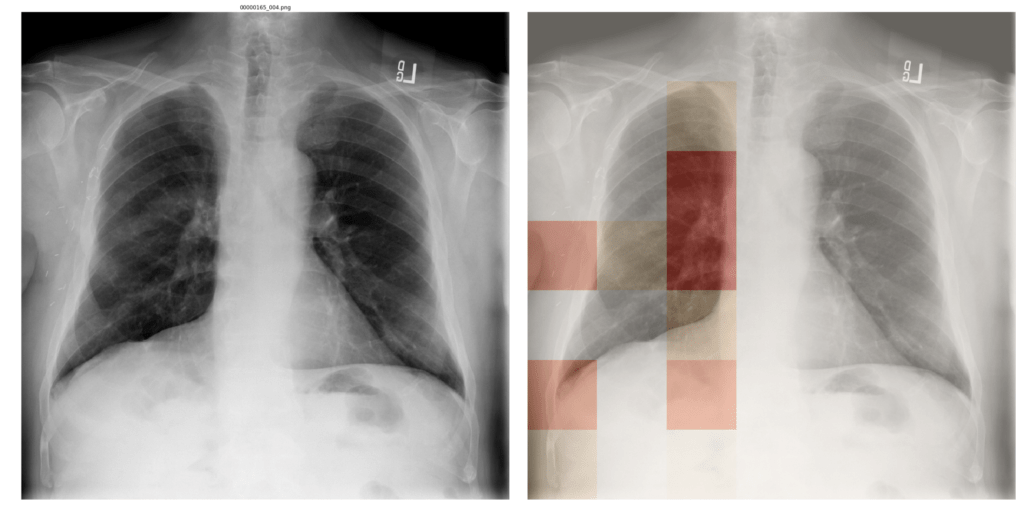
Figure 1 – Example of Pleural Thickening Detection
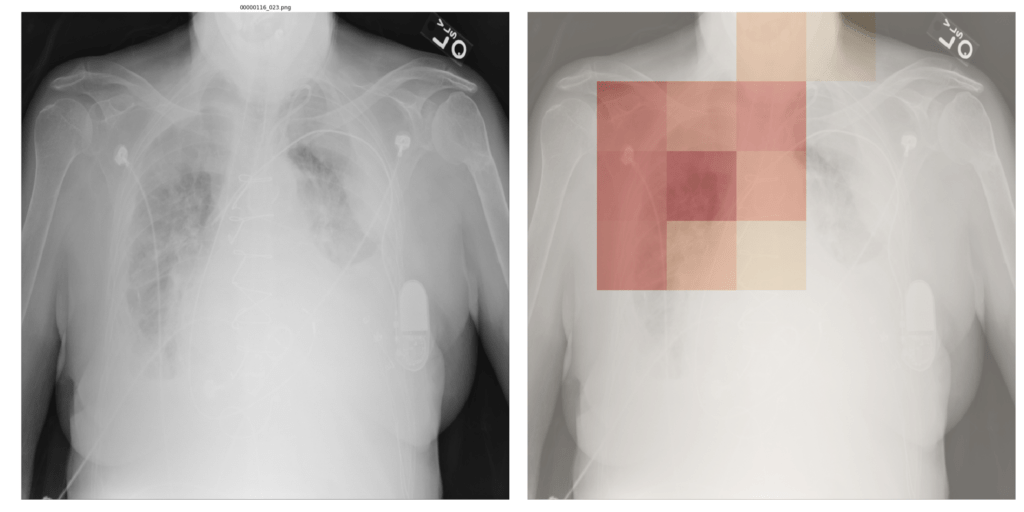
Figure 2 – Pulmonary Infiltration Detection
The team lead of the pitch faction presented the work in a 3 minute presentation followed by 2 minutes of questions format. We were announced as finalists the following day.
As finalists we needed to fill out an application to win the prize – one prize for each cloud provider was allocated – consisting of credits on the cloud we built the proof of concept on. The team drew together to complete the form which detailed the teams plans should they win, the work to do, roadblocks, risks and mitigations, other sources of funding and a timeline to completion. It was around a week later that we were informed that our application had been chosen as the winner for the Amazon Web Services prize.
The team was excited to have won but were more aware than ever on the effort necessary to bring a proof of concept to reality. Medical data is highly sensitive and access is fraught with challenges. Installing software within hospitals highly secure and isolated infrastructure is problematic. Work continues slowly to try and overcome these obstacles and the team persists in trying solving this issue not just for Queensland and Queenslanders but on an international scale.
Thank you to Dr Timothy Cleaver and Dr Sujan Chowdhury of TechConnect IT Solutions for drafting this article for publication.